With the development of high-throughput biological technology, various types of biological omics data have been generated, which offers an unprecedented new opportunity to understand the molecular mechanisms of life. However, how to effectively analyze these data is the challenge that biologists, medical scientists and information scientists have to face now.
Recently, research team led by Dr. Hongqiang Wang in IIM proposed a new high-throughput data meta analysis method. The method combines joint matrix decomposition with a gene regulation model so that the concordance of molecular activities between different genetic molecular levels can be flexibly probed in identifying cancer molecular biomarkers in meta-analysis context. Numeric experiments showed the performance of the proposed method is superior to the previous methods. The study also revealed two major factors that dominantly influence the meta-analysis of omics data, i.e. data dependence and heterogeneity. The two factors could divide the existing methods into three groups that perform significantly different in reproducibility, as shown in the figure below. The real-data application led to finding a number of known or unknown methylation-driven cancer genes for non-small cell lung cancer (NSCLC). These new biomarkers provide novel clues for developing potential NSCLC methylation-targeted drugs.
The study has been recently published by Oxford University Press in the latest issue of Bioinformatics journal. Two anonymous reviewers commented the work as “The jNMFMA utility and stability of the results seem to be impressive”, “…I feel this could be a very useful piece of work.” Dr. Wang’s work is potentially useful in meta-identifying molecular biomarkers in many application research areas like agriculture and medicine.
Please check the detail of the article at
http://bioinformatics.oxfordjournals.org/cgi/content/abstract/btu679?ijkey=HAtiDUYB3vPECYl&keytype=ref
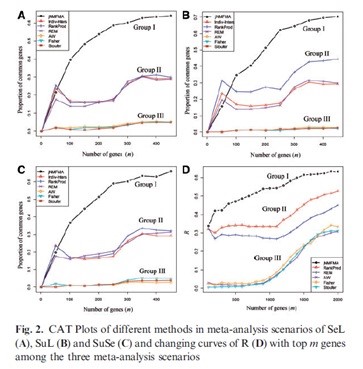